Research:Understanding thanks
Background[edit]
The thanks feature is a tool with which editors can quickly and easily give each other positive feedback. This feature was first introduced to the English Wikipedia on May 30th, 2013 and has now been implemented across all projects. It is well-documented; thanks are recorded on project specific logs, and editors can ask about the feature on the talk pages. Yet, despite the feature being well-established, little research has been done on it. This is problematic as Wikimedia can only exist as a public trove of information through the immense continual effort of editors, placing community health and motivation boosting initiatives such as wiki-thanks in a key role. The aim of this project, therefore, is to better understand the thanks feature: its scope, the characteristics of a typical thanks interaction, and the effects of receiving thanks on individual editors.
A search for previous work on the thanks feature revealed the Nemoto, Okada 15 paper, [1] which examines differences in thanks usage across languages. Our work was informed by this study, and parts of this project build on its analyses. The Harburg, Matias 14 research, [2] which draws a contrast between the thanks feature and wiki-love, was useful as well.
Our work was further informed by a literature search which suggested that positive external motivation (rewards, recognition) can lead to an increase in contribution to a community. It is well-established that "people will socially loaf less and contribute more to a group the more they like it". [3] A positive community environment, therefore, would both give editors a better experience and increase edit activity. A positive environment may actually be one of the most crucial elements for increasing engagement, as “social and cognitive factors seem to be more important than issues of usability in predicting contribution to [a] site". [4] These social factors can have a powerful impact, as "external incentives do, in fact, affect a contributor’s internal incentives and goal setting... performance feedback and social recognition are often the most economical choices for OC [online community] reinforcement". [5] Positive recognition being so impactful implies that the thanks features has the potential to significantly increase both community well-being and editor motivation and activity.
Results[edit]
Overview[edit]
Our analysis is separated into two parts: understanding how people interact with the thanks feature and assessing the impact the feature has on editor motivation and engagement. In part one, we compile data on general use, such as the characteristics of the feature’s users, how likely different types of editors are to receive thanks, etc. We also establish a link between receiving a thank and having a higher edit count. This helps contextualize our results from part two, in which we, with a reasonable degree of confidence, prove this link to be causal, at least for edit count in the short-term. We discuss our results at a high-level below.
Discussion[edit]
1A: Thanks Usage
The scope of the thanks feature, or the number of editors the feature has touched since it was first introduced, is generally within the 4-6% range in the larger languages. In the set of editors with 5+ edits, the scope of the feature is 15-17%, indicating the existence of a small group of active editors who are responsible for the vast majority of thanks.
Language Thanks Givers Thanks Receivers Editors % Thanks Givers % Thanks Receivers German 23433 31567 390603 6.0 8.08 Spanish 14079 13924 526009 2.68 2.65 Italian 8742 9412 186733 4.68 5.04 Portuguese 8093 8593 194509 4.16 4.42 Polish 5880 6506 83949 7.0 7.75 Farsi 4611 4829 108114 4.26 4.47 Dutch 4704 4830 89006 5.29 5.43 Arabic 6873 6662 148112 4.64 4.5 Korean 1855 1874 68285 2.72 2.74 Thai 1045 610 29780 3.51 2.05 Norwegian 1171 2500 41501 2.82 6.02
- Table 1: Scope of thanks feature in select languages
The thanks feature is not as widely used as we would hope, but it has become more prevalent in recent years. Even in languages where editor count has dropped, thanks usage rates have increased. The ubiquity of increasing usage rates suggests editors that may have only recently become aware of the feature. If this is the case, efforts to increase exposure would have significant benefits.
Language Thanks Givers 2018 Thanks Givers 2016 % Thanks Givers 2018 % Thanks Givers 2016 Italian 1910 1511 6.03 4.98 Portuguese 1273 1314 5.16 4.75 Polish 1297 940 8.67 6.66 Farsi 1103 575 5.72 4.47 Dutch 935 915 6.82 6.28
- Table 2: Thanks usage rates
Note: Data was collected in 6-month intervals (Jan-July as opposed to Jan-Dec).
The distribution of thanks across different editor groups highlights a significant disparity between novice and experienced editors. We compare the average number of thanks received by a set of editors per month or day to the average number of thanks received by the same editors per month or day counting only months or days in which they received at least one thank. We do not include editors who have never received a thank, and we separate the data into novices (bottom 20% of editors by edit count) and experienced (top 20% of editors by edit count). The editors we studied received thanks in groups more often than they would have if thanks were given at random times, and there was a strong correlation between those who were given more thanks and those who had high edit counts.
Language Sample Thanks in Year Thanks in Month Thanks in Day Italian Bottom 20% 2.69 1.68 1.18 Italian Top 20% 119.62 13.07 1.59 Portuguese Bottom 20% 2.95 1.98 1.34 Portuguese Top 20% 206.24 22.22 2.33 Polish Bottom 20% 2.34 1.63 1.19 Polish Top 20% 48.63 6.3 1.42 Farsi Bottom 20% 2.73 1.91 1.28 Farsi Top 20% 123.0 13.74 1.74 Dutch Bottom 20% 2.37 1.48 1.11 Dutch Top 20% 81.0 9.61 1.5
- Table 3: Distribution of thanks for individual editors
This thanks to edit count correlation is further corroborated by our analysis of average thanks received over all editor percentiles. The study reveals that the top 5% of editors receive the most thanks by far, though they receive the least thanks in comparison to their edit counts. This skew is even more apparent in the graphs for thanks given.
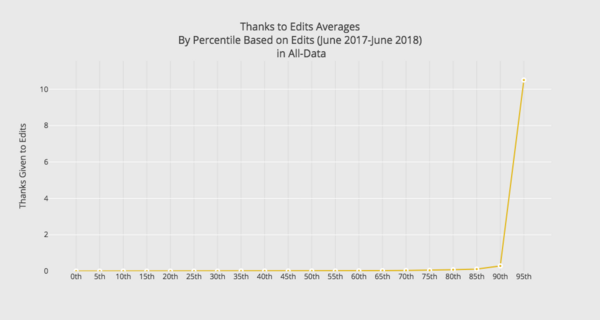

To preserve brevity, a number of sub-projects were left out of this report. A more comprehensive analysis and code pipelines are linked for anyone interested in seeing the full project. This includes:
- the thanker network and the thanks feature's scope
- an analysis of the percentage of editors responsible for various percentages of thanks
- a comparison of thanks senders vs receivers
- the distribution of thanks on an individual level, ie how clustered vs spread out thanks are
- the rankings of all projects by thanks usage rates
- a breakdown of thanks received by editor experience
We have linked project meeting notes as well.
1B: Editor Dropout
The editor dropout study is more relevant to the general research community than to this project specifically. This study defines a threshold number of months of inactivity after which an editor can be considered to have left a project entirely. To define this, we compute the probability of an editor who has been inactive for x months returning in month x+1, and we conduct a Markov chain analysis to verify our results.

The matrix above describes the expected number of months an editor will spend in each state given the state they are currently in. The states represent the number of months for which an editor has been inactive, with AE, or active editor, corresponding to 0.
We suggest a threshold of 3 months based on both this matrix and our initial analysis, though we discuss other alternatives here.
2: Motivation
In part two, we attempt to prove a causal link between receiving a thank and having a high edit count, demonstrating that thanks can increase an editor's activity, at least in the short-term. To do this, we match editors who received a thank on some day with editors who did not receive a thank on some (potentially different) day and compare their subsequent edit activity. Because we only match between editors with similar characteristics, we can be reasonably confident that the thank, and not some other factor, caused the future edit count differences we saw.
The following is an example result from the Polish Wikipedia:
Group Tenure Edits Thanks Short-term Edits Short-term Thanks Next Day's Edits Editors with Higher Counts Thanked 2848.3 555.3 5.8 56.8 0.3 9.6 46 Unthanked 3021.7 573.1 5.9 60.6 0.3 5.7 15
- Table 4: Motivation study results sample
We found a positive correlation between the five features (tenure, edits, thanks, short-term edits, short-term thanks) and the dependent variable, future edit count. That is to say, as the value of any of the five features increases, the expected future edit count increases as well. In the table above, the unthanked editors have a higher average for every feature. We would therefore expect them to have a higher average future edit count as well. This is not reflected in the data, suggesting that the difference we see in future edit count is caused by the one field along which the groups are not balanced: whether an editor has just been thanked.
It is possible that the edit count discrepancy above is caused by some unaccounted for confounding variable, but our predictive test results suggest that this is not the case. A random forest classifier we trained, for example, demonstrated that the features we chose have reasonable predictive power. Another test revealed that a variety of random features either had less weight on the overall prediction than the selected five or led to similar matchings.
For anyone who would like to read more or verify our results, we have linked the code used to run this study as well as the full details of the other tests we ran.
Conclusions[edit]
We conclude with reasonable confidence that thanks strongly impact short-term editor activity. We do not prove the existence of long-term effects, but we would posit that they exist and are impactful. Because the feature is largely used by a group of editors who are already highly active and committed, each individual thank is unlikely to have this far-reaching impact, but the effects of thanks may compound over time, and receiving a thank as a new editor could potentially be transformative to a Wikimedia career. While the long-term effects of thanks were not determined in this study, our findings indicate that increasing thanks feature usage would positively affect editor retention and activity. We would not want thanks to become so common as to be meaningless, but the feature is far from that point, if it exists, and the findings of this study show that (a) the majority of the editor population has yet to receive a thank and (b) receiving a thank can significantly impact an editor's activity, at least in the short-term. It is our belief, therefore, that engaging more editors with the thanks feature would be beneficial to editor motivation, activity, and possibly retention.
Presentations[edit]
References[edit]
- ↑ Wiki Thanks: Cultural Differences in Thanks Network of Different-Language Wikipedias (Nemoto, Okada 15) Retrieved 6/4/18
- ↑ The Effect of Appreciation on Wikipedians (Harburg, Matias 14) Retrieved 6/4/18
- ↑ Using Social Psychology to Motivate Contributions to Online Communities (Ling et al. 06) Retrieved 6/5/18
- ↑ Motivations to Participate in Online Communities (Lampe et al. 10) Retrieved 6/5/18
- ↑ Motivating Content Contributions to Online Communities: Toward a More Comprehensive Theory (Tedjamulia et al. 05) Retrieved 6/5/18